Neural networks will help manufacture carbon nanotubes
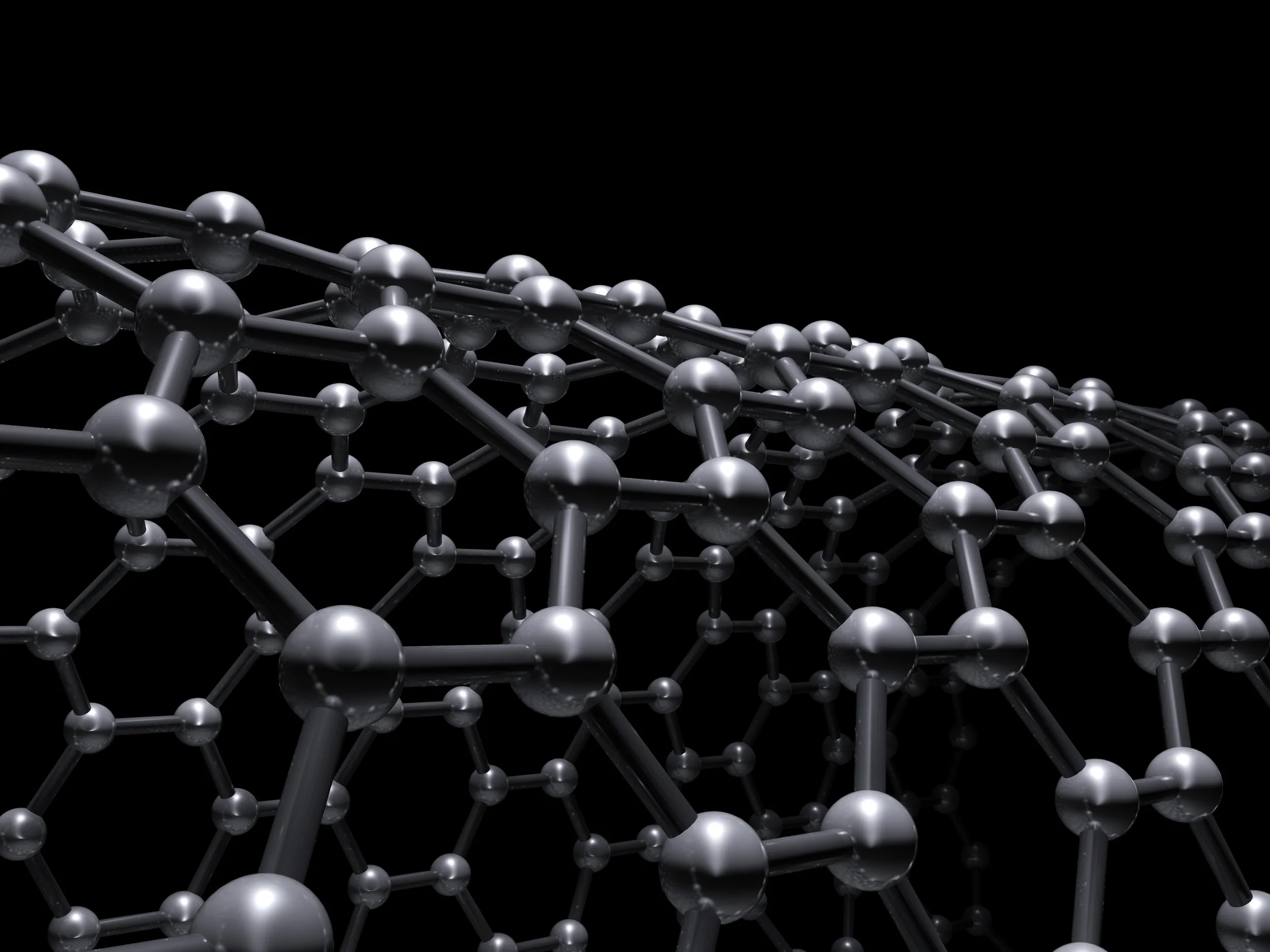
Thin films made of carbon nanotubes hold a lot of promise for advanced optoelectronics, energy and medicine, however with their manufacturing process subject to close supervision and stringent standardization requirements, they are unlikely to become ubiquitous anytime soon.
"A major hindrance to unlocking the vast potential of nanotubes is their multiphase manufacturing process which is extremely difficult to manage. We have suggested using artificial neural networks (ANN) to analyze experimental data and predict the efficiency of single-walled carbon nanotubes synthesis," explains one of the authors of the study and Skoltech researcher, Dmitry Krasnikov.
In their work published in the prestigious Carbon journal, the authors show that machine learning methods, and, in particular, ANN trained on experimental parameters, such as temperature, gas pressure and flow rate, can help monitor the properties of the carbon nanotube films produced.
"The development of human civilization and the advancement of the materials manufacturing and application technologies are closely interlinked in the era of information and technology, with both materials and computational algorithms and their applications shaping our day-to-day life. This is equally true for ANN that have evolved into an indispensable tool for dealing with multi-parameter tasks, which run the gamut from object recognition to medical diagnosis. Over the last 25 years, little headway was made in the development of electronics based on carbon nanotubes due to the complex nature of the nanotube growth process. We believe that our method will help create an effective carbon nanotube production framework and open new horizons for their real-life applications," says Head of Skoltech's Laboratory of Nanomaterials, Professor Albert Nasibulin.