Advancements in Solid-State Electrolytes for Safer and More Efficient Batteries
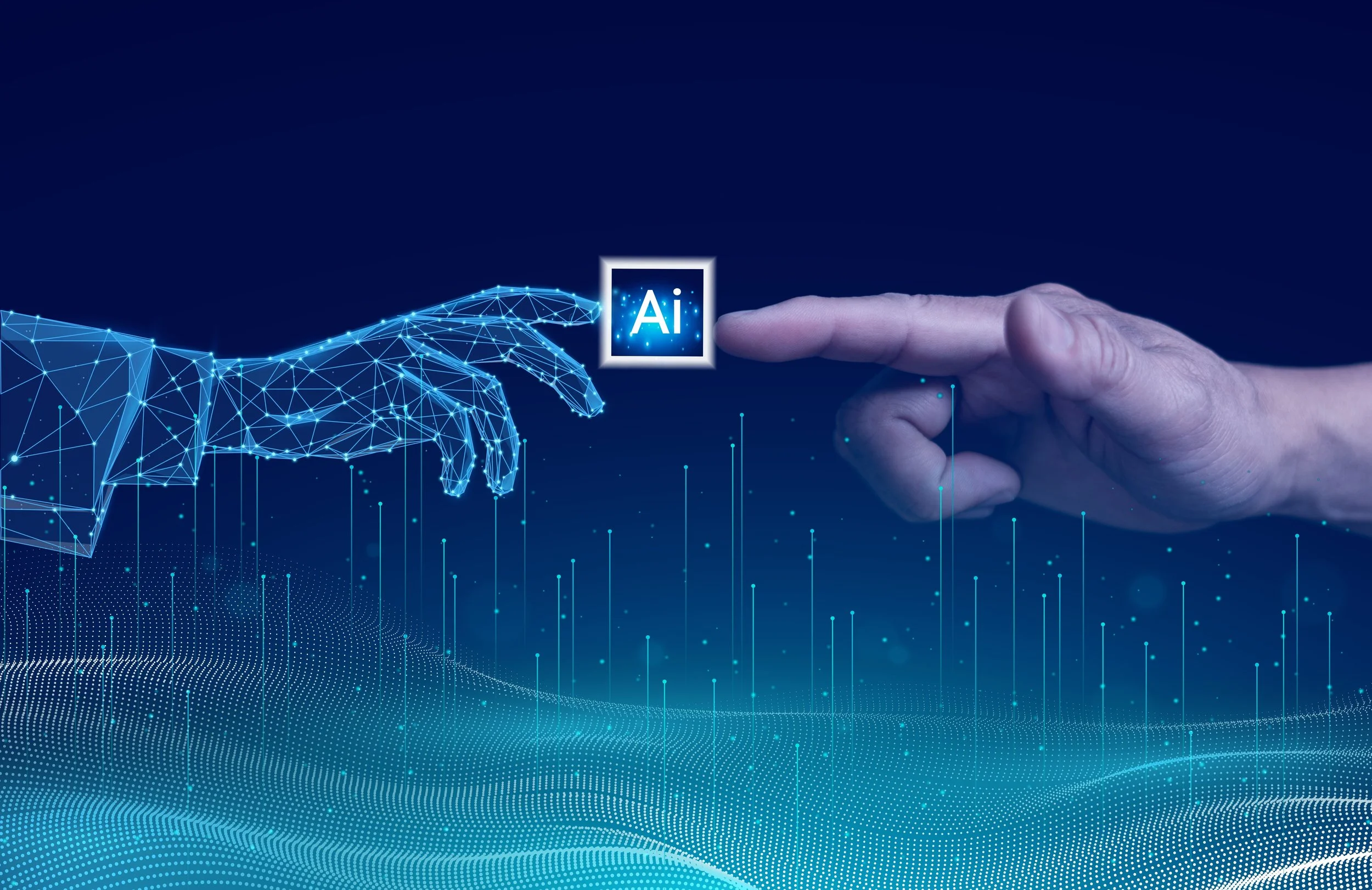
As society moves towards cleaner energy solutions, the transition to electric vehicles and renewable energy sources like wind and solar is becoming increasingly vital.
However, with this shift comes the growing risk of battery fires. To mitigate this risk and enhance battery performance, the next generation of batteries will likely rely on new solid-state electrolytes. Research in this area has been challenging due to the sheer number of material options and parameters involved, but advancements in machine learning are providing a much-needed solution.
A team of materials scientists has developed a dynamic database of hundreds of solid-state electrolytes, leveraging artificial intelligence techniques to direct research more effectively. Their innovative approach is detailed in a paper published in the journal Nano Materials Science on September 10, 2023.
Organic solvents are commonly used as electrolytes in many rechargeable batteries. These substances, usually in liquid or gel form, facilitate the movement of ions between the positive and negative electrodes. While organic solvents provide good conductivity and efficient ion transport, they pose significant safety and performance risks. Researchers have been seeking alternative electrolyte materials for some time.
Organic solvents can be flammable and may lead to thermal runaway reactions, causing fires or explosions. Additionally, they are prone to chemical decomposition, which can result in gas formation and the breakdown of the electrolyte over time, reducing the battery's performance and lifespan. They also sometimes suffer from a limited range of voltages that the battery can operate within.
One promising alternative is all-solid-state batteries (ASSBs), which replace the traditional liquid or gel organic solvent with a solid electrolyte. This not only eliminates the risk of leakage and explosion but also offers higher energy density and potentially faster charging times. However, discovering solid-state electrolytes (SSEs) with high ionic conductivity—the ability for ions to move through the battery and produce a current—has been fraught with challenges due to their complex structures and the relationship between those structures and performance. Until now, only SSEs with sluggish ion migration have been identified, hindering the development of ASSBs.
"Making matters worse is the sheer number of SSEs to choose from," said Hao Li, a materials scientist with the Advanced Institute for Materials Research at Tohoku University and the corresponding author of the paper. "There are hundreds of possibilities, and it's a real challenge for researchers to tackle such a volume of options while keeping track of the many various parameters of optimal performance."
To address this, the team created an experimental dynamic database, the Dynamic Database of Solid-State Electrolytes (DDSE). Initially, it contained over 600 potential SSE materials, covering a wide range of operating temperatures and encompassing various cations and anions. This database was designed to explore the relationships among different variables.
A dynamic database is one that can be easily updated and modified frequently, allowing for real-time changes and additions to the data it contains. In this case, the DDSE is continuously updated with new experimental data, receiving weekly updates. By January 2024, it had grown to include over 1000 materials.
Researchers then applied machine learning to the DDSE to overcome the limitations of human analysis and the high computational costs of theoretical calculations. Without machine learning, researchers struggled to handle the large atomic systems of SSEs and the complexity of the chemical reactions involved.
By leveraging machine learning, researchers can make better predictions about novel solid-state electrolyte materials at much lower computational and financial expense, saving time compared to previous trial-and-error methods of SSE design. This approach has allowed them to uncover intricate relationships among various variables, including ion transport, composition, activation energy (the energy required to initiate a chemical reaction), and conductivity. These insights are enabling the development of new guidelines for SSE design.
The team has already identified development and performance trends of SSEs across various material classes, as well as performance bottlenecks for each class. Furthermore, the DDSE was designed with a user-friendly interface, allowing other battery and materials scientists to update and utilise it themselves, fostering broader collaboration in the field.
In summary, as the world increasingly adopts electric vehicles and renewable energy, the need for safer and more efficient battery technologies becomes critical. The development of a dynamic database of solid-state electrolytes, combined with the application of machine learning, represents a significant step forward in overcoming the challenges of battery research. By making better predictions and uncovering key performance trends, scientists are paving the way for the next generation of safer, high-performance batteries.
Author
Isabella Sterling
Content Producer and Writer